Introduction
Artificial Intelligence (AI) has the potential to transform healthcare by providing accurate and efficient patient care. However, one of the significant challenges in implementing AI in clinical settings is generalisation. Generalisation refers to the AI system’s ability to apply its knowledge to new data that may differ from the original training data. A recent comment published in npj Digital Medicine explores the challenges of generalisation in clinical AI and discusses potential solutions to ensure trustworthy patient outcomes.
Understanding Generalisation in Clinical AI
In healthcare, generalisation is crucial for AI systems to make accurate predictions across diverse patient populations. Unfortunately, many machine learning (ML) models struggle to generalise effectively. This is particularly problematic in clinical settings, where the stakes are high. For instance, ML models trained on biassed or non-representative datasets may fail to provide reliable predictions for underrepresented groups.
One reason for this challenge is the inherent complexity and variability of clinical data. Clinical datasets are often high-dimensional, noisy, and contain numerous missing values. These factors can lead to overfitting, where the model performs well on training data but poorly on new, unseen data. Moreover, societal biases reflected in training data can exacerbate algorithmic biases, leading to poorer generalisation for certain groups.
Selective Deployment: An Ethical Approach
To address the generalisation challenge, recent work in bioethics advocates for the selective deployment of AI in healthcare. Selective deployment suggests that algorithms should not be deployed for groups underrepresented in their training datasets due to the risks of poor or unpredictable performance. This approach aims to safeguard patients from unreliable predictions while ensuring that AI systems are used responsibly.
Case Study: Breast Cancer Prognostic Algorithm
Breast cancer predominantly affects biological women, with a 100:1 ratio compared to biological men. Consequently, men experience worse health outcomes and are underrepresented in clinical datasets. A recent breast cancer prognostic algorithm, trained solely on female data, accurately predicts outcomes for women but is expected to underperform for men. Excluding men from using this algorithm protects them from unreliable predictions but raises ethical concerns about fairness and equal access to advanced treatments.
Technical Solutions for Generalisation
To improve the generalisation of AI models in clinical settings, several technical solutions can be employed: Data augmentation involves adding real or synthetic data to training datasets, enhancing the model’s ability to learn from diverse examples. Fine-tuning large-scale, generalist foundation models on limited data or using training paradigms like model distillation and contrastive learning can boost generalisation in low-data scenarios. Out-of-distribution (OOD) detection methods flag samples that significantly deviate from the training data, identifying cases where model predictions may be unreliable. Additionally, involving a human-in-the-loop in medium- and high-risk clinical applications provides an extra layer of safeguarding, ensuring critical decisions are not solely dependent on AI models.
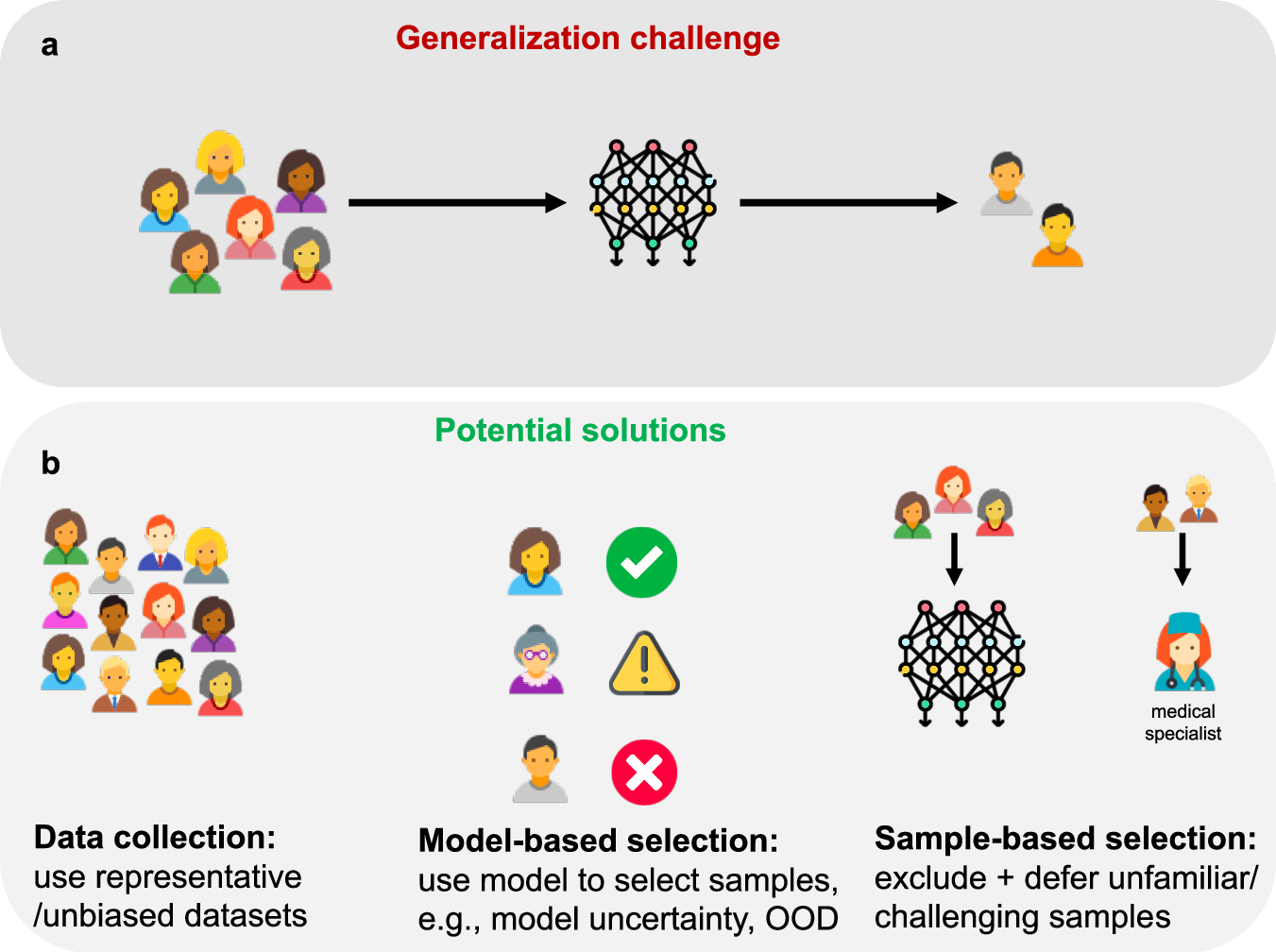
Figure 1. AI generalisation challenges and solutions
Ethical Considerations and Future Directions
Ethical considerations are crucial in the deployment of AI in healthcare to ensure fairness and equal access to advanced treatments. Selective deployment, supported by robust technical solutions, can help balance these ethical concerns. Active data-centric AI techniques are essential for guiding data collection and valuation, ensuring that training data is representative and diverse, thereby reducing algorithmic biases. Additionally, synthetic data generation can enhance model generalisation by augmenting small datasets and simulating real-world distribution shifts, but it must be done using fair generation approaches to avoid propagating biases.
Conclusion
Generalisation remains a key challenge for the responsible implementation of AI in clinical settings. Selective deployment and techniques like data augmentation, model distillation, and OOD detection enhance AI model reliability. Ethical considerations must guide these efforts to ensure that all patients benefit from advanced AI-driven healthcare solutions.